SML group at ETH
Department of Computer Science, ETH Zurich.
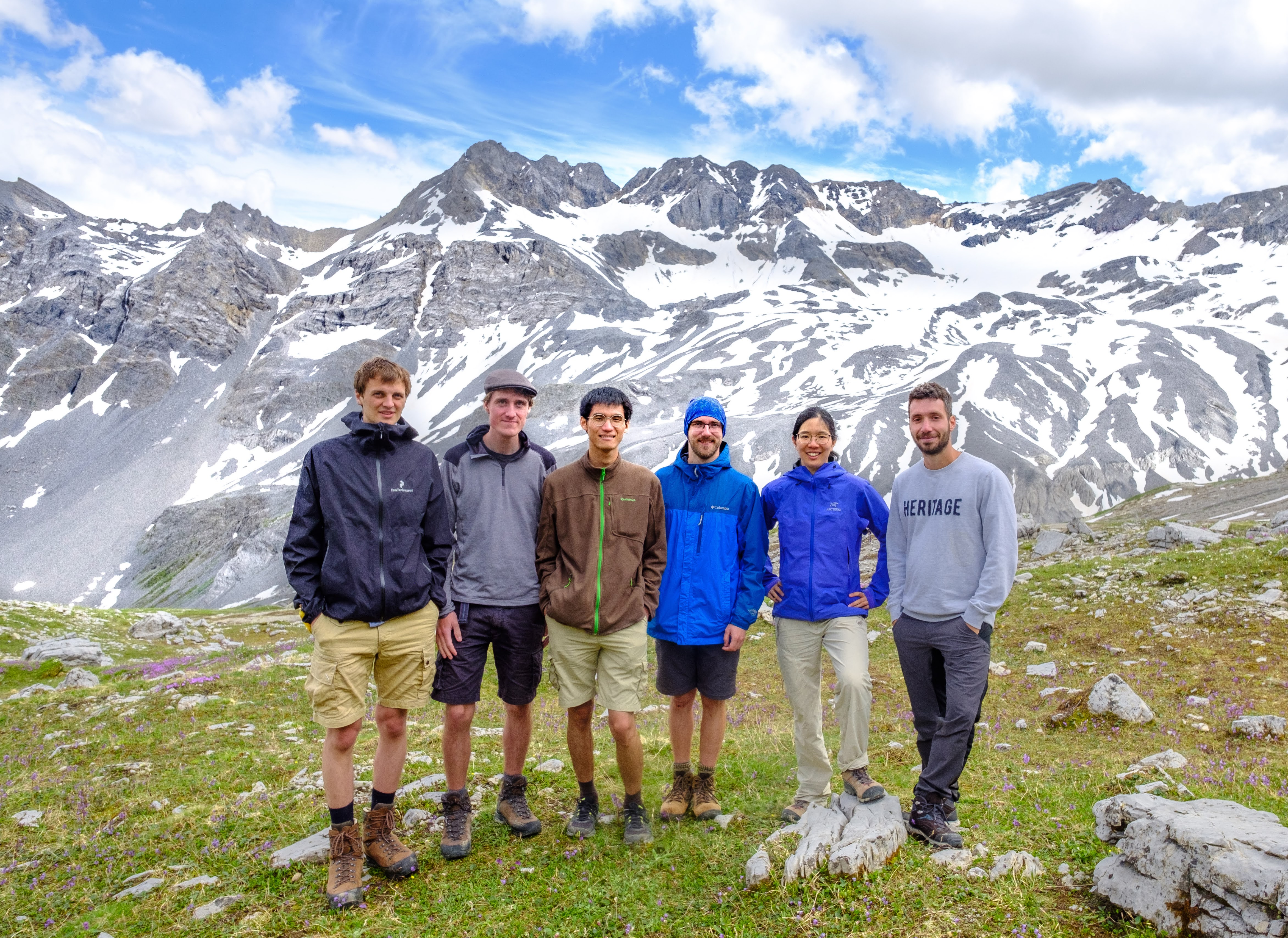
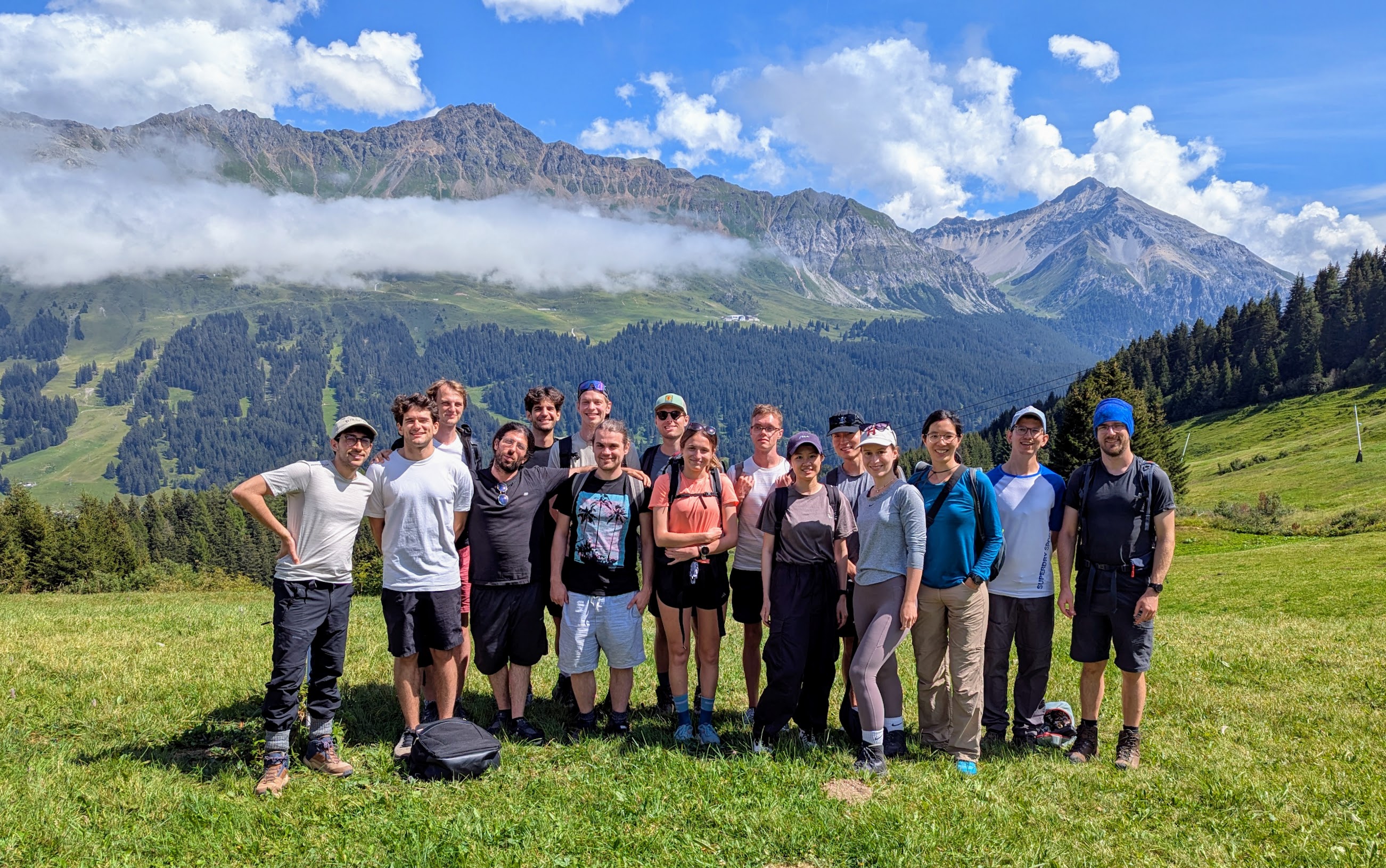
Welcome to the website of the Statistical Machine Learning group at ETH Zurich!
We are a group of curious minds from different parts of the world who study exciting questions in the intersection of statistics and machine learning. On a high level, we like to develop theoretical understanding for methodological advancements and vice versa.
Most projects in our group revolve around trustworthy modern machine learning including robust inference against attacks during training and test time, generalization of overparameterized models, as well as questions arising around sample-efficient causal inference and privacy preservation of sensitive data. We are also exploring the fundamental limits, methodologies and trade-offs between different notions of trustworthiness such as (train and test-time) robustness, privacy and fairness. Please have a look at our recent papers to get a better sense for our research interests.
news
Jan 23, 2025 | All of our three recent submissions got accepted at the 2025 editions of AISTATS and ICLR: towards better copyright protection, on doubly-robust treatment effect identification using multiple environments and multi-objective learning in high dimensions! |
---|---|
Dec 9, 2024 | Many of us spent some time this semester at the Simons Institute Berkeley, participating in the Long-term program Modern Paradigms in Generalization, where Fanny gave a tutorial on benign overfitting and a talk on robust mixture learning with large outlier proportion. She was also the workshop chair of the final workshop Unknown Futures of Generalization with a panel on the future of scientific research in the age of AI and a debate on how scaling current LLMs could be enough to prove open math conjectures. |
Jul 23, 2024 | Andrii, Alex and Nicolo all successfully defended their PhD thesis in the last half year with flying colors. We wish them all the best for their future endeavors! |
Jan 20, 2024 | Four of our recent submissions got accepted at the 2024 editions of AISTATS, SaTML and ALT. On privacy: Effective semi-supervised semi-private learning, private data synthesis for sparse queries; On causal inference: quantifying hidden confounding; On overparameterization and interpolation: max-l1-margin classifiers |
Dec 10, 2023 | Thanks to the crowd who showed up at our Neurips Tutorial on overparameterization! You can still watch the video and find more information on the tutorial website. Also gave a talk at ICSDS Lisbon on a line of work we just started on quantifying hidden confounding with additional datasets. |